Recently we published a comprehensive paper on virus detection, including classification and quantification via machine learning, based on surface enhanced Raman scattering spectroscopy (SERS), in Biosensors and Bioelectronics. The title of the paper is "Rapid and quantitative detection of respiratory viruses using surface-enhanced Raman spectroscopy and machine learning."
In the paper, we report the detection of thirteen respiratory virus species including SARS-CoV-2, common human coronaviruses, influenza viruses, and others. Virus detection and measurement have been performed using highly sensitive SiO2 coated silver nanorod array substrates, allowing for detection and identification of their characteristic SERS peaks. Using appropriate spectral processing procedures and machine learning algorithms (MLAs) including support vector machine (SVM), k-nearest neighbor, and random forest, the virus species as well as strains and variants have been differentiated and classified and a differentiation accuracy of >99% has been obtained. Utilizing SVM-based regression, quantitative calibration curves have been constructed to accurately estimate the unknown virus concentrations in buffer and saliva. This study shows that using a combination of SERS, MLA, and regression, it is possible to classify and quantify the virus in saliva, which could aid medical diagnosis and therapeutic intervention.
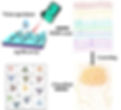