Diagnosis of SARS-CoV-2 infection allows for disease intervention, control, and management for COVID-19. The real-time reverse transcriptase-polymerase chain reaction (RT-PCR) is considered the gold standard used to detect the virus. Due to the high testing volumes, the process has a long turnaround time, i.e., 2-3 days. It also requires expensive equipment and involves highly trained staff. Other fast diagnostic methods, such as lateral flow assay based on antibody detection, have limitations such as lower specificity and sensitivity. Thus, there is a critical need for a rapid and low-cost point-of-care (POC) diagnostic method to accurately diagnose SARS-CoV-2 infections in patients.
Recently, our group has developed three rapid, portable, and cost-effective methods to detect SARS-CoV-2 in human nasopharyngeal swab (HNS) specimens using surface enhanced Raman spectroscopy (SERS) and deep learning: RNA hybridization, ACE-2 capture, and direct detection.
For the RNA hybridization method (see ACS Sensors 8, 297 - 307 (2023)), the SERS sensor was prepared using a silver nanorod array (AgNR) substrate by assembling DNA probes to capture SARS-CoV-2 RNA. SERS spectra of HNS specimens were collected after RNA hybridization, and the corresponding SERS peaks were identified. The RNA detection range was determined to be 103 - 109 copies/mL in saline sodium citrate buffer. A recurrent neural network (RNN)-based deep learning model was developed to classify 40 positive and 120 negative specimens with an overall accuracy of 98.9%. For the blind test of 72 specimens, the RNN model gave 97.2% accuracy prediction for the positive specimens, and 100% accuracy for the negative specimens. All the detections were determined in 25 min.
For the ACE2-based sensor (see ACS Sensors 9, 3158 – 3169 (2024)), the sensor is based on ACE2 functionalized SiO2-coated silver nanorod arrays (AgNR@SiO2), and SERS spectra with concentrations from different variants were collected. After an appropriate spectral pre-processing, a deep learning algorithm, CoVari, is developed to predict both the viral variant species and concentrations. Using a 10-fold cross-validation strategy, the model achieves an average accuracy of 99.9% in discriminating between different virus variants and R2 values larger than 0.98 for quantifying viral concentrations of the three viruses, demonstrating the high quality of the detection. The LOD of the sensor is determined to be 10.472 PFU/mL, 11.882 PFU/mL, and 21.591 PFU/mL for SARS-CoV-2, SARS-CoV-2 B1, and CoV-NL63, respectively. In an unknown specimen test, classification accuracy can achieve > 90% for concentrations larger than 781 PFU/mL, and the predicted concentrations consistently align with actual values, highlighting the robustness of the proposed algorithm.
For the direct real specimen detection (Advanced Materials Interfaces, DOI 10.1002/admi.202400013), a dataset of 4800 SERS spectra from 120 positive and 120 negative inactivated clinical HNSs are collected directly on the AgNR@SiO2 substrates without any labels. A SFNet algorithm is tailored to adapt to the unique spectral features inherent to SERS data, achieving a test accuracy of 98.5% and a blind test accuracy of 99.04%. Moreover, an optimized SFNet algorithm unveils the capability of estimating SARS-CoV-2 viral loads, accurately predicting the cycle threshold values (Ct values) of the three vital gene fragments—ORF1ab, N gene, and S gene—with a root mean square error (RMSE) of 1.627 (1.3 for blind test). The methodology is substantiated using actual clinical specimens and completed in less than 15 minutes. This work shows that the SERS technology is competitive to RT-PCR.
All these results indicate that SERS combined with deep learning could be a potential rapid POC COVID-19 diagnostic platform.
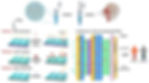