Detecting PFAS in Water at PPT Levels
- Yiping Zhao
- Jul 10, 2024
- 2 min read
High sensitivity PFAS (per- and polyfluoroalkyl substances) detection is critical due to their persistence in the environment and potential health risks. These "forever chemicals" are widely used in industrial applications and consumer products, leading to widespread contamination of water, soil, and air. Even at low concentrations, PFAS have been linked to serious health issues, including cancer, hormone disruption, and immune system impairment. The most common method for analyzing PFASs in environmental samples is liquid chromatography coupled with mass spectrometry (LC-MS) . While this laboratory-based technique offers precise results, it is often associated with high costs and limited sensitivity. Recently, fluorescence detection has shown promising advancements, achieving sub-ppt levels of limit of detection (LOD) and the ability to differentiate between perfluorooctanoic acid (PFOA) and perfluorooctanesulfonic acid (PFOS) . Despite these developments, significant challenges remain, particularly in achieving high sensitivity with the desired LOD, detecting and differentiating multiple PFAS species, and ensuring field applicability.
In a recent publication (Sensors & Diagnostics, 2024, DOI: 10.1039/D4SD00052H), we demonstrated the effectiveness of using Raman and surface-enhanced Raman scattering (SERS) spectroscopies to identify and quantify various PFASs in water. Experimental Raman spectra of different PFASs reveal unique peaks for classification. While direct SERS measurements from Silver nanorod (AgNR) substrates may not show distinct PFAS peaks, PFAS presence on SERS substrates induces significant spectral changes. Integrating these SERS spectra with machine learning (ML) techniques allows for successful differentiation and quantification of PFOA in water, achieving a limit of detection (LOD) of 1 ppt. Modifying AgNR substrates with cysteine and 6-mercapto-1-hexanol enhances SERS-ML capabilities. Despite alkanethiol molecules affecting spectral features, PFAS and PFOS concentrations cause observable spectral variations. A support vector machine model achieves 93% accuracy in differentiating PFOA, PFOS, and references, independent of concentration. A support vector regression model establishes LODs of 1 ppt for PFOA and 4.28 ppt for PFOS, with classification accuracy improving to 95% after removing spectra with concentrations lower than LODs.
This work was mainly conducted by a physics undergraduate student, Mr. Joshua C. Rothstein, and a first year engineering graduate student, Mr. Jiaheng Cui. Details can be found at D4SD00052H (rsc.org).
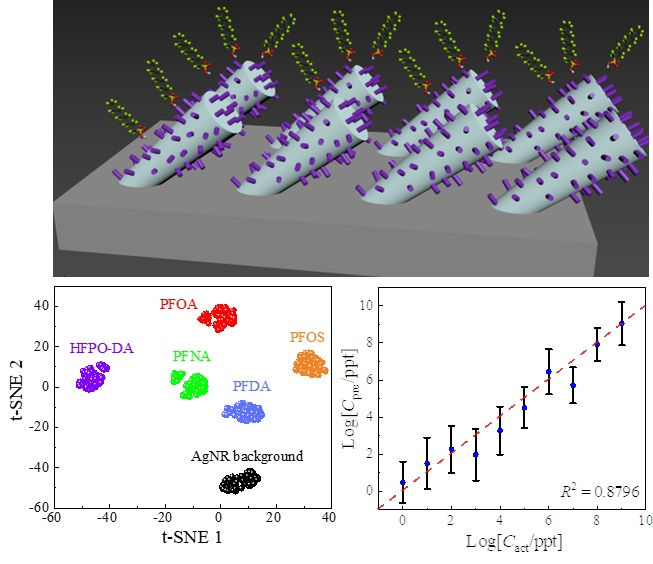
Comments